What is Data Quality
Data quality assesses how effectively a dataset fulfills essential criteria, including accuracy, completeness, validity, consistency, uniqueness, timeliness, and integrity. This assessment is vital to all data governance initiatives within an organization.
The Significance of Data Quality in Artificial Intelligence
AI systems depend significantly on the quality of the data inputted into them; thus, the caliber of this data directly affects their effectiveness and accuracy. High-quality data ensures that AI models function optimally, yielding accurate predictions and delivering reliable insights that businesses can confidently utilize for informed decision-making.
Nevertheless, prevalent data quality issues—such as incomplete datasets, duplicate records, inaccuracies, and outdated information—can significantly affect the outcomes of artificial intelligence initiatives. For instance:
- AI models trained on incomplete data may develop biases or fail to capture the full scope of variables necessary for accurate predictions.
- Duplicate data can skew analysis results, leading to inefficient or erroneous decisions.
- Outdated information can result in recommendations that are no longer relevant, potentially costing businesses valuable time and resources.
- Inconsistencies in data, where different systems store similar data in conflicting formats, can further complicate data integration and analysis, making it challenging for AI systems to provide cohesive insights.
Addressing these issues is vital to avoid “garbage in, garbage out” scenarios, wherein subpar input data results in unreliable outputs. Consequently, upholding rigorous standards of data quality is not only advantageous but essential for effectively harnessing AI throughout business operations.
Data Preparation for AI
Effective implementation of artificial intelligence relies heavily on careful data preparation. Thoroughly collecting, cleansing, and curating your data establishes a solid foundation for harnessing advanced AI capabilities, which in turn facilitates informed decision-making and strategic actions.
Data Collection and Preprocessing
The foundation of any AI project is comprehensive data collection. Acquiring data from a variety of sources enhances the dataset, thereby offering a more extensive foundation for AI to learn from and draw inferences.
Preprocessing is an essential phase in which raw data is refined for analysis. Data profiling plays a crucial role in identifying anomalies or missing values that could potentially distort results. For example, absent data in customer purchase histories may result in erroneous conclusions regarding buying preferences. The preprocessing stage encompasses various tasks, including normalizing data—scaling it within a specific range—addressing missing values through imputation, and detecting outliers that may signify either data entry errors or legitimate anomalies.
Data Cleansing and Classification
Data cleansing is essential for ensuring the reliability of your datasets. This process involves correcting inaccuracies and eliminating duplicates, both of which are vital for maintaining the integrity of your AI models. Clean data ultimately results in more accurate and effective analytics.
Data classification involves assessing the sensitivity and significance of information within an organization. In a corporate context, data can be categorized into public datasets, which can be widely shared, and confidential datasets, which necessitate stringent access controls. This classification process is essential for implementing appropriate security measures and ensuring compliance with data protection regulations.
Data Transformation and Validation
Transforming data for AI readiness might involve aggregating sales data to a suitable granularity or developing new features based on existing data, like calculating the lifetime value of customers based on their purchase history. This step is vital for preparing the data in formats that AI models can efficiently use.
Validation follows transformation to ensure the data maintains consistency and quality. This often involves checking for data integrity and consistency across different data stores to ensure that the transformation rules have been applied correctly.
Metadata, External Data Sources, and Storage Solutions
Metadata is integral to AI data management, offering vital information regarding the origin, purpose, and structure of the data. This information is essential for effective data handling and utilization.
Integrating external data sources—such as market trends, demographic information, and economic indicators—can greatly augment the predictive capabilities of AI systems. Additionally, the transition to cloud-based storage solutions facilitates scalable, flexible, and cost-effective data management, which is essential for processing the substantial volumes of data generated in today’s landscape.
Data ethics and compliance are of utmost importance, particularly when managing sensitive information. It is vital to ensure that data usage adheres to legal standards, such as the General Data Protection Regulation GDPR, in order to safeguard consumer privacy and foster trust.
Understanding and Curating Your Datasets: A Comprehensive Guide
Being deliberate in the selection of datasets is essential. Recognizing and employing various dataset types—such as categorical and numerical data—along with understanding their specific characteristics, enhances the design of more effective AI models. Furthermore, identifying and addressing gaps within datasets is critical for comprehensive analysis and for preventing biased decisions that may arise from incomplete data.
This thorough methodology for data preparation—encompassing collection, cleansing, transformation, and additional steps—guarantees that your AI systems are established on a foundation of quality and integrity. Such an approach not only improves performance but also ensures the delivery of reliable and actionable insights.
Harnessing the Potential of Artificial Intelligence through Quality Data
With your data now clean and meticulously organized, your organization is ideally situated to harness the transformative potential of artificial intelligence. High-quality data not only enhances the efficiency of AI operations but also paves the way for a multitude of opportunities to integrate AI across various business processes.
With this foundation, you can leverage artificial intelligence to enhance various functions, including predictive analytics, automated customer service, and intelligent automation processes. These AI-driven solutions significantly improve decision-making, streamline operations, and personalize customer interactions, ultimately resulting in enhanced profitability and increased customer satisfaction.
For instance, we collaborated with to develop an intelligent and personalized AI sales outreach assistant. This innovative tool enables business development and sales team members to engage in deeper and more meaningful communications with prospects, customers, and partners. I encourage you to explore the complete case study for further insights.
Underwater Datasets For AI
The transition from thorough data preparation to the implementation of AI is critical for any organization seeking to fully leverage technology to improve its operations. High-quality data serves not merely as a prerequisite but as a vital catalyst for unlocking advanced AI capabilities. These capabilities have the potential to revolutionize your business landscape by streamlining operations, enhancing customer interactions, and driving sales performance.
Our underwater datasets are meticulously produced to integrate seamlessly with your AI models, unlocking personalized benefits that enhance your company’s efficiency and competitiveness.
Recommended for you
- AI
Công cụ gán nhãn dữ liệu là yếu tố thiết yếu trong quá trình phát triển...
- News
Hãy tưởng tượng bạn có thể ghi lại thế giới thực — từng khúc quanh của...
- Datasets, Computer vision, Machine learning, News
In the fast-moving landscape of computer vision, the accuracy and reliability of machine learning models depend...
Coral Mountain Data
Office
- Group 3, Cua Lap, Duong To, Phu Quoc, Kien Giang, Vietnam
- (+84) 39 652 6078
- info@coralmountaindata.com
Data Factory
- An Thoi, Phu Quoc, Vietnam
- Vung Bau, Phu Quoc, Vietnam
INDUSTRY
RESOURSE
COMPANY
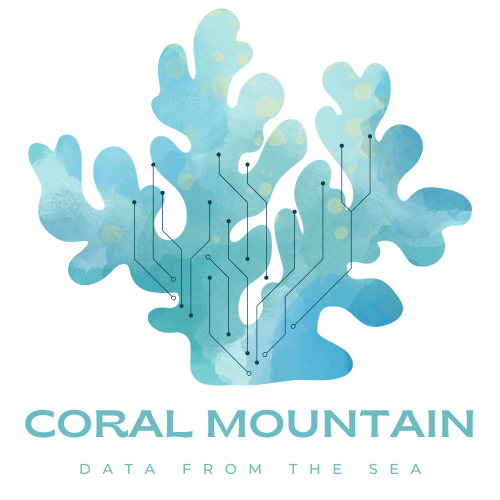
Copyright © 2024 Coral Mountain Data. All rights reserved.